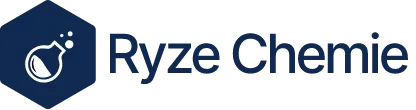
Stay up-to date on the
latest blogs. Join our
newsletter today!
This site is protected by reCAPTCHA and the Google Privacy Policy and Terms of Service apply.
What Is The Difference Between Accurate Data And Reproducible Data
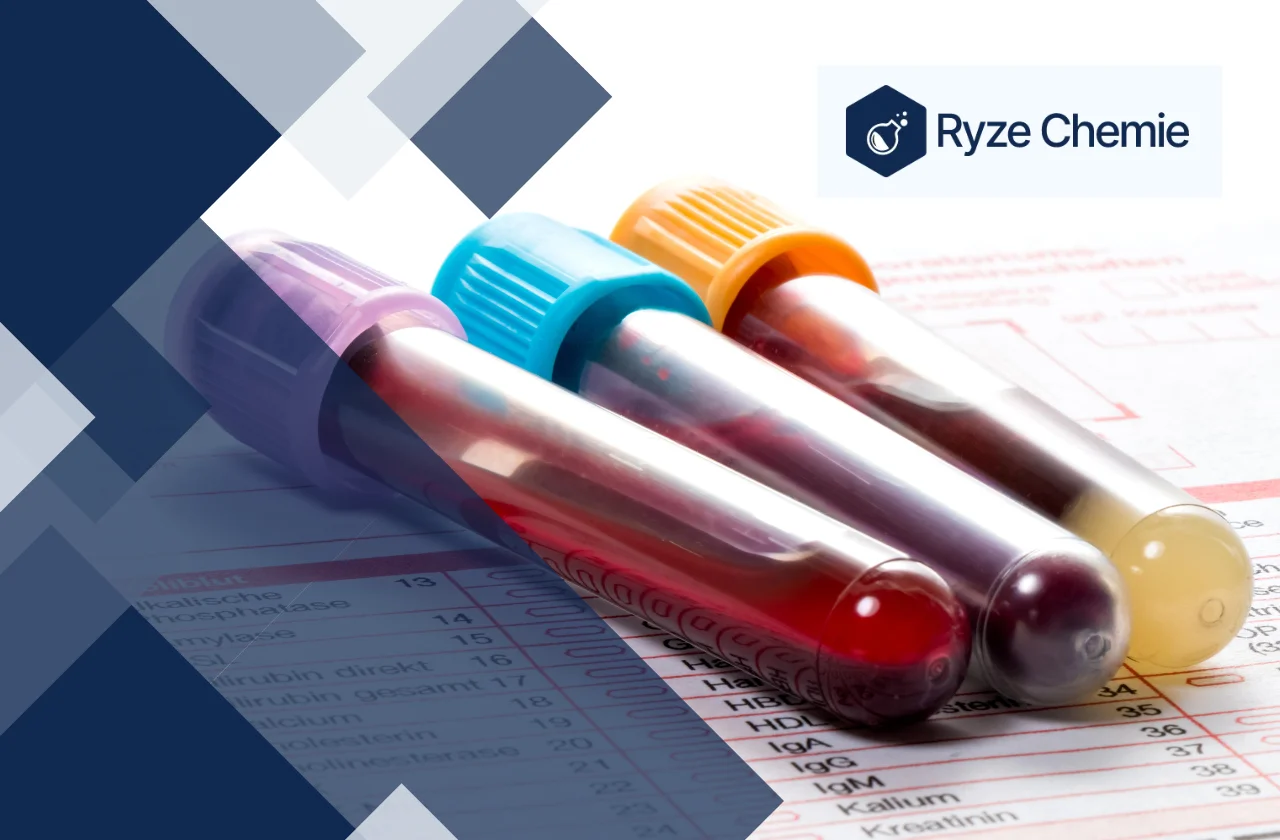
13 mins read · May 15, 2024
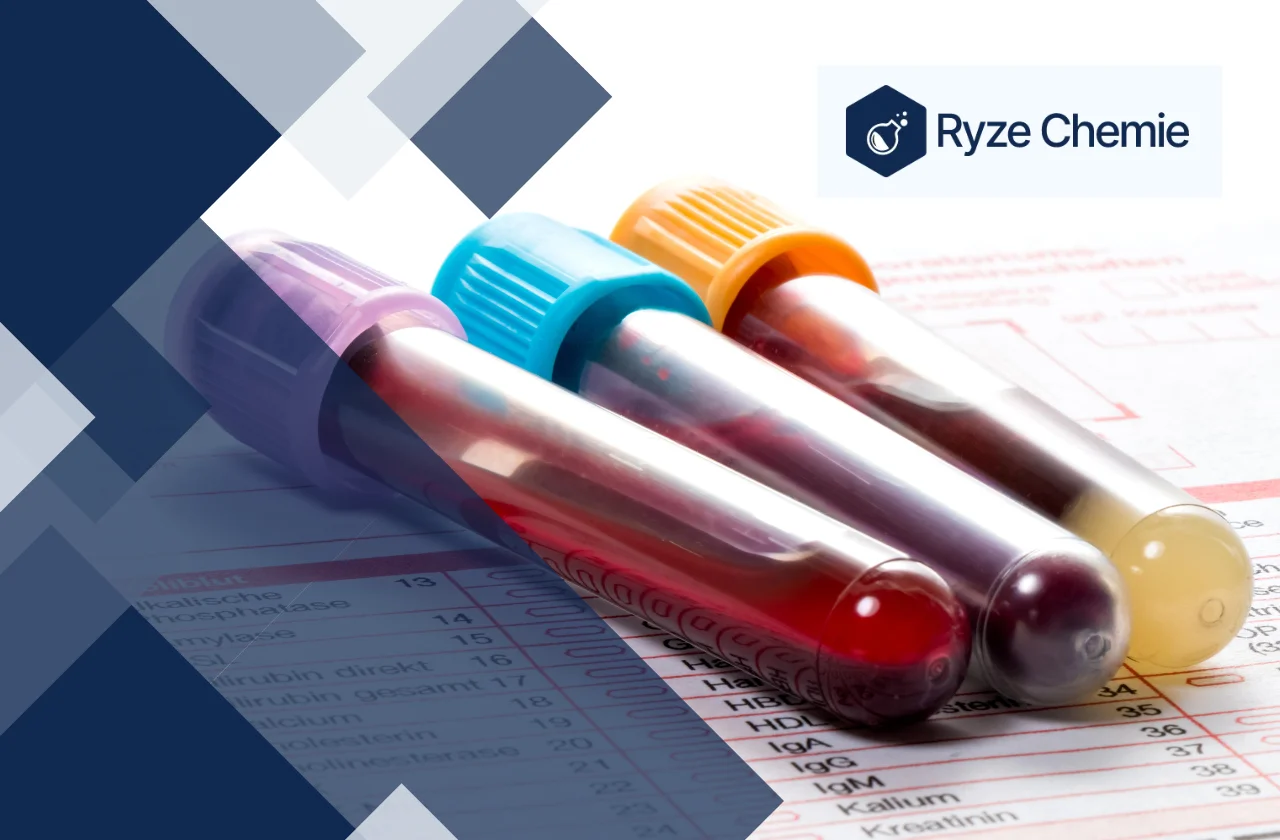
In labs, confusion regularly arises between accurate and reproducible records. This confusion can cause mistakes in experiments. Lab personnel need clarity on those ideas to make certain dependable actions.
Accurate data displays how close measurements are to the authentic value. Reproducible records means getting consistent results when repeating a test under the same conditions. Understanding this distinction is essential for keeping the integrity of research.
In this article, we will delve deeper into those fundamental concepts, providing practical insights and strategies tailor-made to enhance the quality and reliability of data generated in chemical laboratories, in the end fostering reliable scientific conclusions and improvements.
What is Accurate Data?
The quality of the results of any laboratory test depends on the accuracy of your data. Accuracy refers to how close the measurement is to the true value of what you are trying to measure.
Imagine measuring the weight of a chemical. The true value is the true weight of the chemical, and your measurement (obtained from a balance) is considered accurate if it is very close to this true value.
However, perfect accuracy is nearly impossible. Errors can affect measurements. Two main types of errors are discussed: systematic errors and random errors.
Systematic Errors:
Systematic errors consistently cause your measurements to deviate from the true value in the same direction – either overestimating or underestimating it. These errors are like a constant bias that skews your results.
Several factors can introduce systematic errors in the laboratory. Here are some common examples:
- Faulty calibration: If your equipment, like a balance or thermometer, is not calibrated correctly, it will consistently provide readings that are slightly off. For instance, an improperly calibrated balance might always read weights 0.1 grams higher than the true value.
- Contamination: If your chemicals or equipment become contaminated with impurities, these impurities can interfere with the measurement. For example, if your measuring flask is not perfectly clean and contains traces of another chemical, the weight of your sample will be higher than expected.
- Incorrect technique: Using the wrong technique when performing an experiment can introduce a systematic error. For instance, if you don't rinse your graduated cylinder before measuring a liquid, you might consistently underestimate the volume due to leftover residue from the previous measurement.
The impact of systematic errors can be significant. They can lead to entirely wrong conclusions if left unchecked. The key to minimizing systematic errors is to:
- Regularly calibrate your equipment according to the manufacturer's instructions.
- Use proper techniques and follow established protocols meticulously.
- Maintain a clean and controlled laboratory environment to minimize contamination.
Example: Systematic Errors in a Chemical Experiment
Let's consider an experiment where you're measuring the concentration of a prepared solution. You prepare a 1 M solution of sodium chloride (NaCl) by dissolving a specific amount of NaCl in water. However, during the preparation, you accidentally use a dirty measuring spoon with salt residue on it. This introduces a small amount of extra NaCl into your solution.
As a result, the actual concentration of your solution is slightly higher than 1 M. Every time you use this solution in an experiment, your results will be skewed because the solution is more concentrated than you intended. This is a classic example of a systematic error caused by contamination.
Random Errors:
Random errors are small, unpredictable variations that occur during measurements. They cause your data points to scatter around the true value, unlike systematic errors which consistently push them in one direction.
Random errors can arise from various factors like:
- Slight differences in how you perform the measurement each time.
- Environmental fluctuations like temperature changes that can affect some measurements.
- Random variations in the equipment itself.
Random errors don't necessarily push your results in a specific direction (up or down), but they do introduce a spread or scattering in your data. The more precise your measurements are, the less scattered your data points will be.
Now, let's explore the concept of reproducible data and its significance in laboratory settings.
What is Reproducible Data?
In chemistry, reproducible data refers to the ability to get the same results when you repeat an experiment under identical conditions. This means that if you follow the same procedures, use the same materials, and conduct the experiment in the same environment, you should expect similar outcomes.
Why is Reproducible Data Important?
Reproducible data is the foundation of reliable research in chemistry. Here's why it matters:
- Validity of Findings: Reproducible data strengthens the validity of your conclusions. If others can repeat your experiment and get the same results, it increases confidence in your findings.
- Comparison and Collaboration: Reproducible data allows researchers to compare results across different studies. This comparison helps build a stronger understanding of chemical phenomena and fosters collaboration between scientists.
- Quality Control: Consistent data is essential for quality control in chemical processes. Reproducible experiments ensure consistent product quality in industrial settings.
Achieving Reproducibility: The Power of Documentation
Detailed and clear documentation is crucial for achieving reproducible data. Here's what you should document:
- Reagents: Specify the exact name, brand, and concentration of all chemicals used. Include lot numbers if relevant. Documenting any purification steps performed on the reagents.
- Procedures: Clearly describe each step of the experiment in chronological order. Include details like quantities, temperatures, reaction times, and mixing methods.
- Equipment: Specify the type of equipment used (e.g., balance, spectrophotometer model). Mention any calibration procedures performed before the experiment.
- Observations: Record all observations made during the experiment, such as color changes, gas evolution, or precipitate formation.
By documenting these details, you create a clear roadmap for anyone repeating your experiment. This ensures they can follow the exact same procedures, increasing the likelihood of getting similar results.
Factors Affecting Reproducibility in the Lab
Several factors can influence the reproducibility of your data in a chemical laboratory. Here are some key ones to consider:
- Variations in Technique: Small differences in how you perform an experiment can affect the outcome. For example, the force used when grinding solids or the speed of stirring a reaction mixture can introduce variability.
- Environmental Conditions: Temperature, humidity, and light exposure can influence chemical reactions. Maintaining consistent environmental conditions throughout the experiment is essential.
- Instrument Calibration: Over time, instruments can drift out of calibration, leading to inaccurate measurements. Regularly calibrating your equipment is crucial for reliable data.
Next, we will examine the relationship between accuracy and reproducibility and why they both matter in chemical labs.
The Relationship Between Accuracy and Reproducibility
Here's where things get interesting. It's possible to have data that is accurate but not reproducible. Imagine a scenario where a faulty balance consistently underestimates the weight of a sample by 5 grams. The data might be accurate in the sense that the underestimation is consistent, but it's not reproducible because repeated measurements won't necessarily yield the same result (always 5 grams less). This is often caused by systematic errors, which consistently bias results in one direction.
Conversely, data can be reproducible but not accurate. Imagine a flawed analytical method that consistently gives the same wrong answer. The results might be reproducible because repeated tests give the same number, but they're all wrong compared to the true value.
The Ideal Scenario: Both Accuracy and Reproducibility
The ideal scenario is to achieve both accuracy and reproducibility. This gives you reliable data that reflects the true value and can be consistently replicated. Here's how to achieve this:
- Regular calibration: Following a strict calibration schedule for your instruments ensures they are providing accurate readings.
- Standardized procedures: Develop and adhere to detailed protocols for each experiment, ensuring consistency across measurements.
- Quality control (QC) samples: Regularly run QC samples with known values to monitor for any deviations in accuracy or precision.
- Good documentation: Maintain meticulous records of all procedures, observations, and results to identify any trends or potential errors.
Now, let's discuss why both accuracy and reproducibility are crucial in chemical laboratories.
Why are Both Accuracy and Reproducibility Important in Chemical Labs?
Chemical laboratories rely heavily on the quality of data generated during experiments. This data forms the foundation for drawing conclusions, developing new products, and ensuring safety. In this critical environment, both accuracy and reproducibility of data are essential for reliable and trustworthy results.
Consequences of Inaccurate Data
Inaccurate data, meaning the measured values deviate from the true values, can lead to a cascade of negative consequences:
- Misleading Conclusions: When measurements are inaccurate, the interpretations drawn from them become skewed. This can lead researchers down the wrong path, wasting time and resources pursuing incorrect hypotheses.
- Failed Experiments: If inaccurate data suggests a successful reaction when it wasn't, subsequent experiments based on this data will likely fail. This not only wastes time and materials but can also discourage researchers and hinder progress.
- Safety Hazards: Inaccurate data related to material properties, reaction yields, or safety limits can pose significant safety risks. For instance, underestimating the reactivity of a compound could lead to an explosion.
Significance of Reproducible Data
Reproducibility refers to the ability to obtain consistent results when the same experiment is repeated. This is crucial because it:
- Verifies Findings: Reproducible data strengthens the validity of the results. If another researcher, following the same protocol, obtains similar results, it increases confidence in the findings.
- Facilitates Collaboration: Reproducible data allows for seamless collaboration between researchers in different labs. When others can replicate the experiment and achieve similar outcomes, it fosters teamwork and the advancement of knowledge.
- Builds Trust in Research: Reproducible data is the cornerstone of trust in scientific research. It assures stakeholders, like funding agencies and regulatory bodies, that the results are reliable and can be used for further development or decision-making.
Real-World Examples
Here are some specific examples highlighting the importance of accurate and reproducible data in different areas of chemistry:
- Analytical Chemistry: In environmental testing labs, accurate data is paramount. If the concentration of a pollutant in water is misrepresented, it can lead to inadequate treatment or even regulatory violations. Reproducibility ensures that these critical measurements are consistent and reliable.
- Pharmaceutical Development: Developing new drugs requires precise measurements of drug properties like potency and purity. Inaccurate data can lead to ineffective or even harmful medications. Reproducibility is essential to ensure that these crucial measurements can be reliably replicated during clinical trials and large-scale production.
- Forensic Chemistry: In criminal investigations, accurate analysis of evidence is vital. Inaccurate data from blood alcohol tests or drug analysis could lead to wrongful convictions. Reproducibility ensures that the evidence analysis methods are reliable and can be consistently applied across different labs.
By prioritizing both accuracy and reproducibility, laboratory personnel can ensure the integrity of their data and contribute to meaningful scientific progress. Moving forward, we'll provide practical tips for ensuring accurate and reproducible data in the lab.
Tips for Ensuring Accurate and Reproducible Data in the Lab
To maintain high standards of data quality, here are some practical tips for ensuring accuracy and reproducibility in chemical laboratories:
1) Calibrate and Maintain Laboratory Equipment Regularly
Laboratory equipment is essential for collecting precise measurements. Inaccurate equipment readings can lead to skewed data and unreliable conclusions. To prevent this:
- Calibrate equipment regularly: Follow manufacturer recommendations for calibration frequency. This ensures your instruments meet the required accuracy standards.
- Maintain a calibration log: Record the date, time, and any adjustments made during calibration. This log serves as a reference and helps track instrument performance over time.
- Perform routine maintenance: Clean and maintain equipment according to the manufacturer's instructions. This minimizes the risk of malfunctions that could affect data accuracy.
2) Implement Good Laboratory Practices (GLP)
Good Laboratory Practices (GLP) are a set of guidelines designed to promote the integrity and reliability of research data. Here are some key GLP principles to follow:
- Label everything clearly: Label all reagents, chemicals, solutions, and glassware with their complete name, concentration, and preparation date. This prevents confusion and potential errors.
- Use proper storage techniques: Store chemicals and reagents according to their specific requirements (e.g., temperature, light sensitivity). This ensures their stability and effectiveness throughout the experiment.
- Document procedures meticulously: Write detailed protocols outlining each step of the experiment, including the amount of chemicals used, reaction times, and specific techniques employed. Clear documentation allows for easy replication of the experiment.
- Dispose of waste safely: Follow established procedures for the safe disposal of chemical waste.
3) Maintain Detailed Laboratory Notebooks
A well-maintained laboratory notebook serves as a permanent record of your experiment. Record the following details:
- Date and experiment title: Clearly identify the experiment for future reference.
- Objective and hypothesis: Briefly state the purpose of the experiment and your predicted outcome.
- Materials and methods: List all chemicals, reagents, and equipment used, including their source and lot numbers. Describe the experimental procedure step-by-step in a clear and concise manner.
- Observations and data: Record all observations made during the experiment, including color changes, gas evolution, or precipitate formation. Include raw data tables and graphs.
- Calculations and analysis: Show any calculations performed on the data and explain the analysis methods used.
- Conclusions: Summarize your findings and discuss whether they support your hypothesis.
4) Analyze Data for Outliers and Inconsistencies
After collecting data, it's crucial to analyze it for potential errors:
- Identify outliers: Look for data points that deviate significantly from the expected trend. Investigate the cause of these outliers. Were there any procedural errors, or do they represent genuine variations?
- Check for inconsistencies: Examine replicate measurements for consistency. If there are large discrepancies, repeat the experiment to identify the source of the error.
- Evaluate data quality: Consider the limitations of the analytical techniques used. Are there alternative methods that might provide more reliable data?
5) Collaborate and Share Data with Your Lab Team
Open communication and collaboration are essential for ensuring data quality:
- Discuss procedures: Before starting an experiment, discuss the protocol with colleagues. This can help identify potential flaws in the design or execution.
- Share data openly: Present your data to colleagues and encourage feedback. This can help identify errors or alternative interpretations.
- Maintain a collaborative environment: Work together to troubleshoot problems and refine experimental techniques. By sharing knowledge and experiences, the entire lab team can contribute to generating high-quality data.
By following these steps, laboratory personnel can significantly improve the accuracy and reproducibility of their data. This not only strengthens the validity of your research but also allows others to confidently replicate your findings and build upon your work.
Conclusion
In conclusion, accurate data reflects precise measurements obtained in experiments, ensuring reliability in results. It provides a solid foundation for scientific conclusions. Reproducible data, on the other hand, enables others to duplicate experimental conditions and achieve similar outcomes. It fosters trust in research findings and supports the validity of scientific claims.
Understanding the distinction between accuracy and reproducibility is crucial for laboratory personnel. Accurate data guarantees correctness, while reproducibility confirms reliability.
Both are essential for advancing scientific knowledge and ensuring the integrity of experimental outcomes in chemical research. Thus, maintaining a balance between accuracy and reproducibility is paramount for credibility in scientific investigations.
Latest Blogs